- Forward Future Daily
- Posts
- š¾ DeepSeek R1: A Model Disrupts the World Market
š¾ DeepSeek R1: A Model Disrupts the World Market
A cost-efficient AI breakthrough ignites geopolitical tensions and market turbulence.
NVIDIA drops nearly 17% as Chinaās cheaper AI model DeepSeek sparks global tech sell-off
In the midst of a global technological upheaval, Chinese AI startup DeepSeek has shaken up world markets with the launch of its latest model, DeepSeek-R1. This development not only raises questions about the future direction of the AI industry, but also about the geopolitical tensions that accompany it.
The company DeepSeek, originally a by-product of the Chinese hedge fund High-Flyer, presented a remarkable model back in December 2024 with DeepSeek-V3. This model impressed with its high efficiency and performance, especially given the limited computing resources used to train it. DeepSeek-V3 was developed using an innovative approach that includes a mixture of expert models (Mixture-of-Experts) and Multi-Head Latent Attention Transformer. The model has a total of 671 billion parameters, of which 37 billion are activated for each query. It was trained on a dataset of 14.8 trillion tokens and achieved top performance in benchmarks such as MMLU and MATH.
Despite a shortage of high-performance computers, DeepSeek has managed to develop an outstanding model that uses significantly fewer specialized chips than comparable Western models. According to reports, DeepSeek-V3 was developed with a budget of just 5.6 million US dollars, while Western competitors such as OpenAI spend over 5 billion US dollars a year. Nevertheless, DeepSeek's reported 5.6 million US dollars must be viewed with caution. On the one hand, this sum presumably relates exclusively to the pure training costs of the model and does not imply infrastructure such as the salaries of the scientists; on the other hand, there is no proof of the low costs so far, but are based solely on statements.
However, this efficiency has led to speculation. Alexandr Wang, CEO of Scale AI, claimed that DeepSeek has around 50,000 NVIDIA H100 GPUs, which casts doubt on the company's claims about the resources used. He made this claim in an interview with the Wall Street Journal in Davos at the World economy forum.
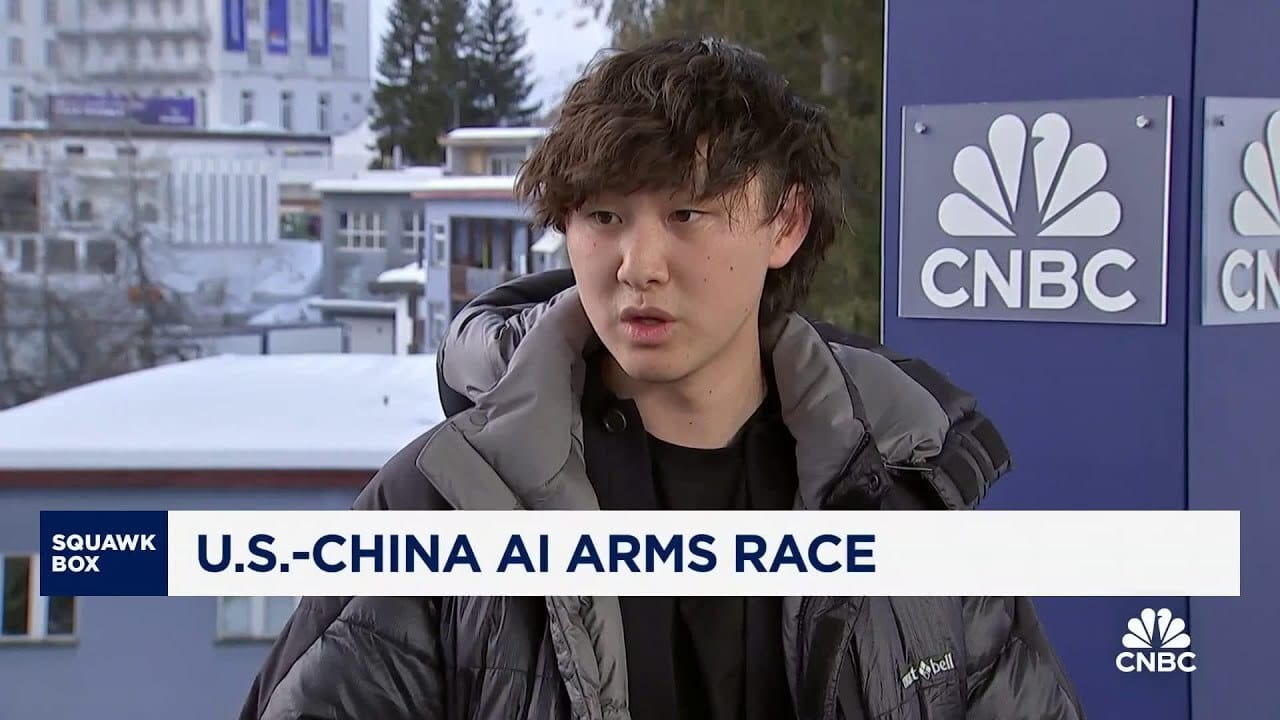
The release of DeepSeek-R1 has reignited the debate about the need for major investment in AI infrastructure. While the US recently announced the āStargateā project with investments of USD 500 billion to secure its leadership position in AI, DeepSeek's approach shows that significant progress can be made even with limited resources. This divergence has led to turbulence on the stock markets, with technology stocks in particular recording significant losses.
These developments are not just technical in nature, but also reflect a geopolitical power struggle in which technological advances are increasingly seen as instruments of national strength.
What Makes DeepSeek-R1 So Special?
What makes DeepSeek-R1 particularly remarkable is its ability to achieve comparable performance to the best reasoning models with significantly fewer resources. While Western companies such as OpenAI invest billions in the development of their models, DeepSeek has managed to create a model that is equivalent in many areas with far fewer resources. This efficiency was achieved through the use of reinforcement learning and an innovative model architecture.
Another key advantage of DeepSeek-R1 is its open source availability under the MIT license. This enables developers worldwide to freely use, adapt and further develop the model, which promotes innovation and the spread of AI technologies.
In terms of technical performance, DeepSeek-R1 shows impressive results in various benchmarks. In AIME 2024, an advanced math reasoning test, it scored 79.8%, outperforming OpenAI's o1-1217, which scored 79.2%. On the MATH-500, which covers a variety of high school-level math problems, DeepSeek-R1 also scored 97.3%, ahead of o1-1217, which scored 96.4%. These results underline the strength of DeepSeek-R1 in complex mathematical tasks.
The model is also impressive in the area of programming. In the Codeforces benchmark, which evaluates algorithm and programming skills, DeepSeek-R1 achieved a percentile rank of 96.3%, just behind o1-1217 with 96.6%. In SWE-bench Verified, a test for software engineering tasks, DeepSeek-R1 scored 49.2%, slightly ahead of o1-1217, which scored 48.9%. These results show that DeepSeek-R1 is capable of handling complex programming tasks effectively.
Another advantage of DeepSeek-R1 is the lack of usage restrictions. Unlike some proprietary models that impose usage restrictions or fees, DeepSeek-R1 can be used without such restrictions. This facilitates integration into various applications and promotes broad acceptance in the community.
In addition, the efficiency of DeepSeek-R1 provides the ability to run the model locally with sufficient computing resources. This means that organizations and individuals can run the model on their own systems without relying on external services, which increases both control over the data and adaptability.
A completely different feature is revealed when you look at the research papers published by DeepSeek. DeepSeek-R1 was created as part of a multi-stage development process in which the use of synthetic data played a central role. The basic DeepSeek-R1 Zero model served as the starting point and was trained exclusively using reinforcement learning (RL) in a unique approach. This approach made it possible to develop complex thinking and reasoning skills, but also presented challenges: the generated output was sometimes difficult to read and there were language mixes that limited user-friendliness.
To overcome these shortcomings and at the same time further improve performance, a multi-stage training process was applied to DeepSeek-R1. In the first phase, the model was fine-tuned with a small amount of carefully selected data to improve the clarity and readability of the output. This was followed by another intensive reinforcement learning training session aimed specifically at strengthening logical thinking and reasoning skills.
A decisive step was the integration of synthetic data. DeepSeek-R1 Zero independently generated a large amount of data, which was then used to fine-tune the model. This innovative process made it possible to combine the advantages of reinforcement learning with data-driven optimization. The result was a model that could not only provide precise and understandable answers, but also set new standards in areas such as mathematics, programming and logical thinking.
In sum, the release of DeepSeek-R1 has reignited the discussion about the need for large investments in AI infrastructure due to all of these features. While the US commits $500 billion to its "Stargate" initiative to secure dominance in AI, DeepSeekās strategy underscores how groundbreaking advancements can arise from resource-efficient approaches. This sharp contrast has fueled volatility in stock markets, with tech shares particularly bearing the weight of investor uncertainty.
Crisis on the Western Stock Market
DeepSeek-R1 is AIās Sputnik moment
The release of DeepSeek-R1 has shaken the technology world and caused considerable turbulence on the global stock markets. It was trained and runs on lower cost chips, reducing reliance on high performance chips such as those from NVIDIA. With a development budget of just 5.6 million US dollars, it challenges the billions invested by US corporations.
This development has cast doubt on the valuation of tech stocks that have previously benefited from the AI boom. NVIDIA, whose GPUs were previously considered indispensable for AI systems, saw its share price fall by 17%, which corresponds to a loss in value of around USD 589 billion. European companies such as Siemens Energy and ASML also suffered considerable losses.
DeepSeek shows that powerful AI models are also possible with older chips. This threatens the business model of NVIDIA and other chip manufacturers, who have previously achieved high margins with high-performance technology. The efficiency of DeepSeek-R1 raises questions as to whether the enormous investments in expensive hardware and infrastructure are still justified.
However, some industry experts also see positive aspects in this development. Pat Gelsinger, former CEO of Intel, emphasizes that more efficient AI models could promote the spread of AI technology, which would ultimately also increase the demand for chips. He praises DeepSeek's engineers for their creative solutions to increase the performance of their models with limited resources.
In the long term, DeepSeek-R1 could herald a new industrial revolution as it significantly expands the possible applications of AI. With lower costs and lower energy consumption, artificial intelligence could penetrate many areas that were previously excluded due to high costs.
In this context, Satya Nadella, CEO of Microsoft, rightly referred to the Jevons paradox, which originally comes from economics. It describes the phenomenon that technological efficiency increases do not reduce the demand for a resource, but rather increase it. Applied to the chip market, this means that cheaper and more efficient AI systems such as DeepSeek-R1 will exponentially expand the possible applications of AI. The result? A much broader range of applications and a rapidly growing demand for chips that can handle these new applications.
Andrej Karpathy, a renowned expert in the field of AI, emphasizes that the āappetiteā of deep learning models for computing power is legendary - not only during training, but also during application (inference). Moreover, DeepSeek has systematically used synthetic data in its training process; in particular, a supervised fine-tuning phase with thousands of synthetic examples from the predecessor model DeepSeek-R1-Zero took place before reinforcement learning (RL). Karpathy is therefore absolutely right to focus future chip requirements on the creation of synthetic data and not just on the pure training of the model. The creation of synthetic data could also mean that pre-training becomes more important again, as the importance of the inference phase has recently shifted in the wake of reasoning.
So while DeepSeek-R1 now shows that models can be trained more efficiently, the focus of the computing load is currently shifting increasingly to the inference phase. This means that AI models are dependent on ever faster and more specialized hardware for their application. Companies such as Groq, which specialize in inference chips, are likely to be among the big winners of this development.
The long-term horizon of AI research does not lie in isolated models such as DeepSeek-R1, but in achieving AGI (Artificial General Intelligence) and ASI (Artificial Superintelligence). These goals require enormous computing resources, as they rely not only on better training algorithms, but also on massive scaling in application. Although the cost of developing intelligence is falling, this cost reduction is more than offset by the exponential growth of AI applications. More users, more data and more complex models: The demand for compute will not stagnate - it will probably explode.
The current collapse on the stock market is therefore merely a price correction due to current events. However, DeepSeek must first prove in the long term that it can keep pace with upcoming models such as o3 or, in the long term, AGI without having access to large numbers of modern chips. This cannot be assumed at present.
Although it is to be welcomed that with DeepSeek-R1 we have a model that can run locally at o1 level, the future prospects are anything but clear and bright; on the contrary, general intelligence or superintelligence will drive the demand for chips to great heights, even if the demand for compute per model should fall. This is because it is distribution that will make general availability possible and the use of AI models by billions of people will drive up the demand for chips.
ā
Get more content from Kim Isenbergāsubscribe to FF Daily for free!
![]() | Kim IsenbergKim studied sociology and law at a university in Germany and has been impressed by technology in general for many years. Since the breakthrough of OpenAI's ChatGPT, Kim has been trying to scientifically examine the influence of artificial intelligence on our society. |
Reply